A recent PWC report showed 46 percent of consumers believe AI will harm human populations by taking away jobs, and another 23 percent think the consequences will be closer to global annihilation. The late Stephen Hawking also was not a fan of AI, warning of the dire consequences of losing control of the technology we’ve created. Bill Gates disagrees, saying people “shouldn’t panic” about the future of AI.
What’s the reality behind AI? Should we be afraid, or should we embrace the technology as something that will help humans be more and do more — faster?
Let’s take a closer look at AI and the implications and applications for machine intelligence. What does our Magic 8 Ball tell us about the future of the thinking machine and its impact on our daily lives?
Disclaimer: This is not intended to be legal advice; rather, by being aware of these issues, we can use AI more effectively and responsibly. This content is for informational purposes only; it does not represent any affiliation, endorsement or sponsorship with the third parties listed.
What’s AI got to do, got to do with it?
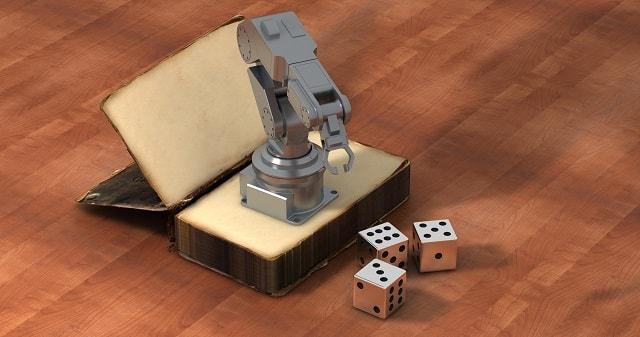
For 60 years, science fiction has been rife with images of self-driving cars, or computers that watch over us like Big Brother. If you’ve been feeling like you’re living in a William Gibson novel, don’t worry — your perception is today’s reality.
AI is the gateway drug to a realm of new business possibilities for everything from marketing to healthcare.
Q: So, what is AI, really?
A: Math. AI is just math.
More specifically, artificial intelligence is a core set of computer processes that allows machines to comprehend, learn, and then react. These “processes” are mathematical and statistical algorithms, along with predictive analysis designed to help a computer learn from its interactions with humans (or another stimulus), incorporate this data, react, and respond according to the new patterns it’s picking up on.
AI is a subset of the computer sciences dating back to the 1950s when it was imagined that computers could carry out tasks in a way that we would consider “smart.” The goal was that the computer would learn from the data itself and not from the rules established by programmers.
My machine isn’t smarter than your 8th grader … yet
History holds up the Turing test as the finish line for an entire realm of science geared toward the pursuit of machine intelligence. Alan Turing was a 1950s mathematics geek-savant who proposed, Computing Machinery and Intelligence, the idea that a machine would be viewed as intelligent when it could fool humans into thinking that it, too, was human.
It was a seminal moment in smart computing, although John McCarthy took credit five years later for the phrase, “artificial intelligence.” MIT and IBM launched projects in foundational AI in those early years, but Turing’s test for computer sentience is still the baseline for defining real machine intelligence.
The Turing test, as it came to be known, required:
- That the computer responds in natural language.
- That it can learn from the conversation by remembering context and prior activity and respond appropriately.
- That it displays “common sense.”
- That it displays emotion by creating something like a poem or music.
Under some of these criteria, it might seem like AI is not only here, but that it’s way smarter than your eighth grader.
However, that isn’t exactly true.
True machine intelligence, or “general AI,” doesn’t exist yet. That’s because all the learning done in current AI models happens in a closed loop designed within certain parameters to fit the machines’ functionary goals. General AI, in theory, achieves technological singularity — the idea that machines evolve to the point where they match and meld with human intelligence to create something completely new.
So, Hal 9000 isn’t here. But we’re getting closer. Google software engineer and futurist, Ray Kurzwell predicts 2029 as the tipping point.
He says the singularity is coming — and many computer scientists agree.
In the past 60 years, AI has experienced the boom and bust of a field rife with controversy. There were periods where funding just didn’t happen; the 1970s are notoriously called the “AI Winter” due to the lack of support for the science.
But we’re in the perfect storm now. It turns out AI needed three primary components to go forth and conquer:
1. Big hardware
Today’s graphics processing units (GPUs) are like Steve Austin the bionic man; they are stronger, better, faster — and they can process images 40 to 80 times quicker than they did just four years ago.
2. Big data
Living in the era of big data fueled by the Internet of Things doesn’t hurt our quest for AI. According to Gartner, there are an estimated 8.4 billion connected “things” out there this year; 2020 will bring us 20.4 billion IoT devices in the market. This cloud-connected hardware is fueling the big data revolution, and a side effect is the acceleration of our quest for AI.
3. Big money
McKinsey confirms that somewhere between $20 billion and $30 billion was dumped into developing proprietary AI in 2016. That’s about three times more than was spent in 2013. A report by Tractica predicts a global market for AI of $59.8 billion by 2025.
More data and faster processing have opened doors previously slammed tight on guys like Turing. That’s probably why Elon Musk is so concerned. We might be very close to true machine intelligence, where the learning processes of computer hardware take off into something we haven’t seen before.
What’s artificial about artificial intelligence?
Some of the core components that will lead us to general AI are being used interchangeably in the popular press. People equate AI with robots, but most AI isn’t housed in a robot chassis — and machine learning isn’t the same as AI.

True artificial intelligence will likely be made up of several programming disciplines. Each of these components is being explored to form whole new subsets of the science, with businesses already benefiting from what we’re discovering.
Consider each of these disciplines as a building block to what might someday lead us to a general AI platform like Hal 9000 or something more benevolent, which hasn’t been conceived:
Machine learning (ML)
This AI subset that works on a system of probability. Based on the data it’s fed, the computer can make statements, decisions, or predictions with a degree of certainty. The addition of a feedback loop, or “recursive algorithms” enables the computer to “learn.”
In this case, learning is simply taking in more data through sensing or being told whether its decisions are right or wrong.
The computer then modifies the approach it takes in the future. Machine learning in applications has improved technologies like facial recognition software to the point where computers are processing at a rate much closer to human cognition. Machine learning is used in some of today’s savvy personal assistants like Facebook’s M, the Amazon Echo — and of course, Apple’s Siri.
Natural language processing (NLP)
It’s a science that allows computers to ferret out the nuances of human language and discern patterns. The translation and speech recognition that comes with your Amazon Alexa have NLP behind its core processes. NLP is computer code based on machine learning algorithms. You see NLP in use every time you hit social media, where companies are actively analyzing consumer behavior patterns by using NLP algorithms.
Neural network
We’re talking about a layered computer architecture designed to identify information in the same way a human brain does. It can be taught to recognize images and classify them according to elements they contain, and then pass the information on to the next neural computing layer. This process is repeated 20 or 30 times until an image is identified. A neural network, like Google Brain, learns through trial and error — kind of like a 2-year-old. Then it rewires itself to handle the patterns in the data it absorbs. This is called deep learning.
Deep learning
This uses the architecture of the neural network.
Deep learning is AI that uses complex algorithms to perform tasks in domains where it learns with little or no human supervision.
Basically, the machine learns how to learn. The concept has been around since 1965, but it hasn’t been until recently that we’ve started seeing breakthroughs in the field. For example, MIT’s Computer Science and Artificial Intelligence Laboratory (CSAIL) has developed a new algorithm that can create a realistic sound to match an action from a video. For example, the computer can see an image of a person using a stick to strike metal and produce a corresponding sound to match. Humans can’t tell the difference between the computer simulated sound and reality.
It’s important to reiterate that these are subsets of the AI discipline, and each has computer scientists and programmers working to expand their knowledge. The result is another subset of AI called cognitive computing, which combines natural language processing, machine learning, neural networks, and deep learning to simulate human thought. The result (so far) is IBM’s Watson, which came to notoriety in 2011 by winning the game show Jeopardy! IBM is working on new ways to harness and monetize Watson. In 2016, they teamed up with H&R Block to change how our taxes get done.
But these cognitive computing systems are designed to augment human capabilities — not replace them — by taking away some of the hard work that comes with data analysis.
Today, these systems process a vast array of data and cull relevant pieces to provide us with actionable insight. They can take the data and “reason” confidently, forming a hypothesis from the underlying concepts. They can converse with humans in natural language, and see, talk and hear.
But something is still missing: your eighth grader can still out-reason and out-create a machine. But the science is getting closer — Google just taught a computer how to dream.
Do androids dream of electric sheep?
Google is investing tons of cash into developing AI technology, and DeepMind is the result. ExtremeTech reports that the latest innovation in DeepMind’s development is that Google is simulating the very human benefits of dreaming by teaching their AI to use the same process.
While there is a lot that we don’t know about the human brain, neurologists posit that human dreams enhance their memory and problem solving. DeepMind would seem to reinforce this theory — “dreaming” has allowed DeepMind to increase its ability to learn 10 times faster. Right now, DeepMind is probably doing a lot of dreaming about computer games. The startling point, though, is that DeepMind is developing original images from real-world objects in a process like what we think our sleeping brains undergo. You can check out what this looks like at Popular Science.
The future state of AI applications
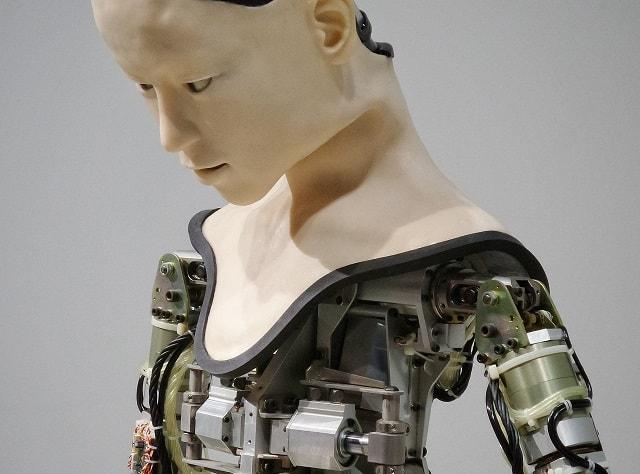
All the pieces are in place for an AI chess move bigger than Deep Blue beating Kasparov. The rulers of the tech industry, from Amazon and Apple to Google, are throwing piles of money into AI research. Computer hardware has evolved, so processing speeds are faster, algorithmic models are getting scarily better, and data from the IoT is exponentially burgeoning.
The only thing that seems to be missing is the C-suite.
McKinsey surveyed more than 3,000 in the C-suite and published their findings in the June 2017 report Artificial Intelligence The Next Digital Frontier? The report concluded:
“…many business leaders are uncertain about what exactly AI can do for them, where to obtain AI-powered applications, how to integrate them into their companies, and how to assess the return on investment in the technology.”
That’s only because the average CEO doesn’t understand that there are more elements of AI’s core components (ML and NLP) entwined in their daily lives than they realize:
- Facebook uses AI in your newsfeed and for photo recognition.
- Microsoft and Apple use AI in Cortana and Siri.
- It’s used for navigation, like on Google Maps and for ridesharing with Uber.
- When you fly, the computers that power autopilot use elements of AI.
- Netflix and Amazon use AI to make recommendations
- The spam filters that block phishing — you bet.
Beyond the science, what has evolved today is the democratization of the technology. Now you too can play with a personal assistant that uses the cruder elements found in AI. There are components of AI in your smartphone; Siri’s algorithms allow her to learn from your behaviors and shift her responses to your queries.
While machine learning and natural language processes are incorporated into many of the cloud and hardware functions we now take for granted, an AI platform hasn’t had a rapid consistent deployment in a monetized business setting. Most pundits agree that is beginning to change.
Can you say, “Hello, Watson?”
H&R Block stood the accounting world on its ear last year by incorporating IBM’s AI, Watson, into tax preparation. Now you can interact with an AI under the supervision of a tax advisor; someday that human element will probably disappear. This has led to fears that the computers will take away jobs. The New York Times suggests the possible displacement of human workers by computers is real:
“We’re not only talking about three and a half million truck drivers who may soon lack careers. We’re talking about inventory managers, economists, financial advisers, real estate agents. What (Google) Brain did over nine months is just one example of how quickly a small group at a large company can automate a task nobody ever would have associated with machines.”
But AI was conceived as a collaboration between computers and their human creators. Accenture says AI could potentially increase human productivity 38 percent by 2035, which will have a profoundly positive impact on the global economy. In fact, they’re predicting that AI could yield, “an economic boost of US $14 trillion across 16 industries in 12 economies by 2035.”
If it doesn’t wipe out humans, first, of course.
Ghost in the machine: What’s next for AI?
Who is currently leading the charge toward general AI and technology singularity?
A March 2017 article in Vanity Fair follows the AI money trail to Google, which spent $650 million to buy DeepMind in 2014. Some startling recent developments in AI research are coming from the “Google Brain” AI team, including:
- Recursive self-improvement algorithms allowed Google’s AI to analyze unlabeled data in 2012.
- Cornell just published a research paper tracking Google Translation’s use of the neural net to radically improve the software.
- Last year a program written by DeepMind beat a human in Go, the world’s most complex board game.
Neural networks are expanding as we write this. Neural networks can now detect tumors in medical imaging more quickly than a human radiologist. But the human brain has somewhere between 100 trillion and 1,000 trillion neurons. The New York Times suggests Google’s current investment in AI will allow them to create the synaptic processes of, say, the brain of a mouse. They point out this important stumbling block in the path of general AI:
“Supervised learning is a trial-and-error process based on labeled data. The machines might be doing the learning, but there remains a strong human element in the initial categorization of the inputs.”
So, the point is while we are currently monetizing elements of AI science in commercial business, we have a long way to go before Elon Musk’s fears of Skynet are realized.
Disclaimer: We're excited to recommend the use of generative AI technology to small businesses, but please be aware that this technology is still in its early stages of development and its effectiveness may vary depending on the circumstances. Additionally, avoid entering sensitive information as AI systems will save your input, and make sure to review the output for accuracy, as it may be incorrect, inaccurate, or out of date.